SoundLib is a year-long project awarded to the Flanders Marine Institute (VLIZ) by the Flemish Government with the aim of creating an underwater sound library of the North Sea for applications in Machine Learning (ML). Specifically, SoundLib will develop a strategy for collecting sound events, design a cost-effective, flexible, and scalable prototype and database architecture, and investigate machine learning methods for automatically processing the underwater sound. In parallel, field recordings of various sound sources will be carried out to populate the underwater sound library.
Sound travels far greater distances in aquatic environments than light does, making it an essential tool for many marine animals when interacting with their surroundings or as a means of communication. All sounds present in the marine environment make up the ‘soundscape’. Natural sound sources can be abiotic (geophony) such as rain, waves, sediment transport, etc., or can be biotic (biophony) such as fish vocalizations, echolocation of dolphins and porpoises, etc. Human presence at sea is ubiquitous and introduces noise (anthrophony) into its environment. Due to the increasing activities, such as shipping, seismic activities, and offshore energy, noise pollution levels are intensifying as well. Specifically in the North Sea, which is a shallow highly exploited sea, the mixture of all sound sources, makes it complex to analyse and understand the soundscape.
The SoundLib project will focus on the long-term underwater recordings in the Belgium part of the North Sea within the LifeWatch project. A focus on all three categories is important and will increase our understanding of the presence and impact of sound on marine life. Well-described sound events will be ingested by the library and available according to the FAIR data principles. To boost the number of sound events per sound category or sound type, machine learning techniques must be applied to efficiently evaluate long-term -and often complex- recordings by automatically detecting and classifying sound, as doing so manually is time-consuming, cumbersome, and prone to human error. Increasing the number of examples per sound type can, in return, be used as input for ML models to detect and classify sound events. During the course of the project, additional recordings will be collected, annotated, and subsequently fed into the library, serving as input data for training and evaluating models. Our goal is to apply these models to long-term recordings of the North Sea to enhance safety of offshore infrastructure and halting certain (illegal) human activities, monitoring its biodiversity and ecological health, and stimulating new research in machine learning and other relevant fields.
Recording, detecting, annotating, and describing sound events involves a large human effort and cost. The SoundLib wants to ease the effort and encourage collaboration amongst scientists by making sound types available as examples as well as input for ML applications.
SoundLib wants to connect to the Global Library of Underwater Biological Sounds (glubs.org), and help increase the knowledge on unknown and known sounds across the world. Providing researchers with the datasets and tools necessary to study the marine environment.
An example of a long-term recording of a soundscape from the shipwreck Birkenfels station (N51°38.940', E2°32.200') in the North Sea containing labelled sound events is as follows:
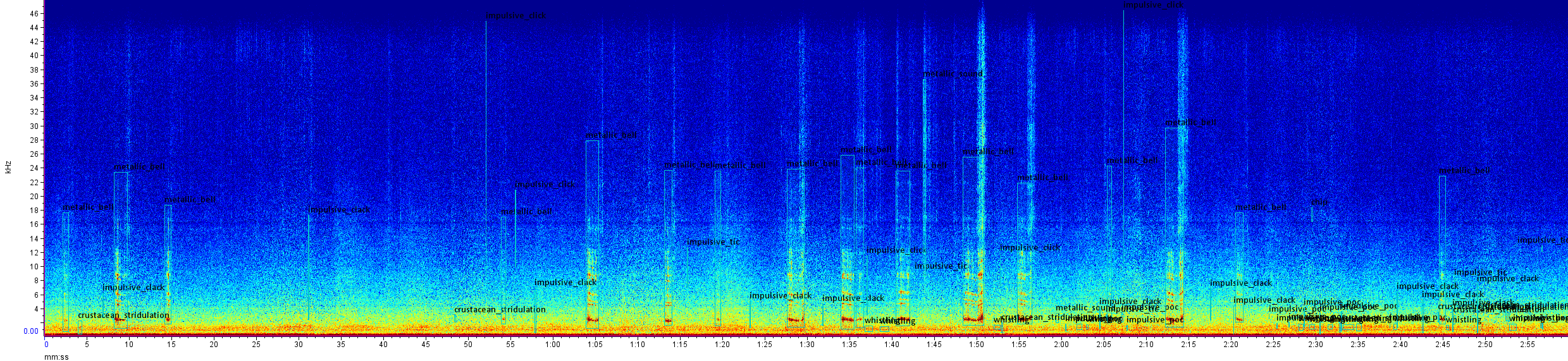
Examples of sound events present in the North Sea are as follows:
Biophony:
Anthropophony:
[1] Bioacoustics Research Program. Raven Pro: Interactive sound analysis software (Version 1.6). (The Cornell Lab of Ornithology, 2014)